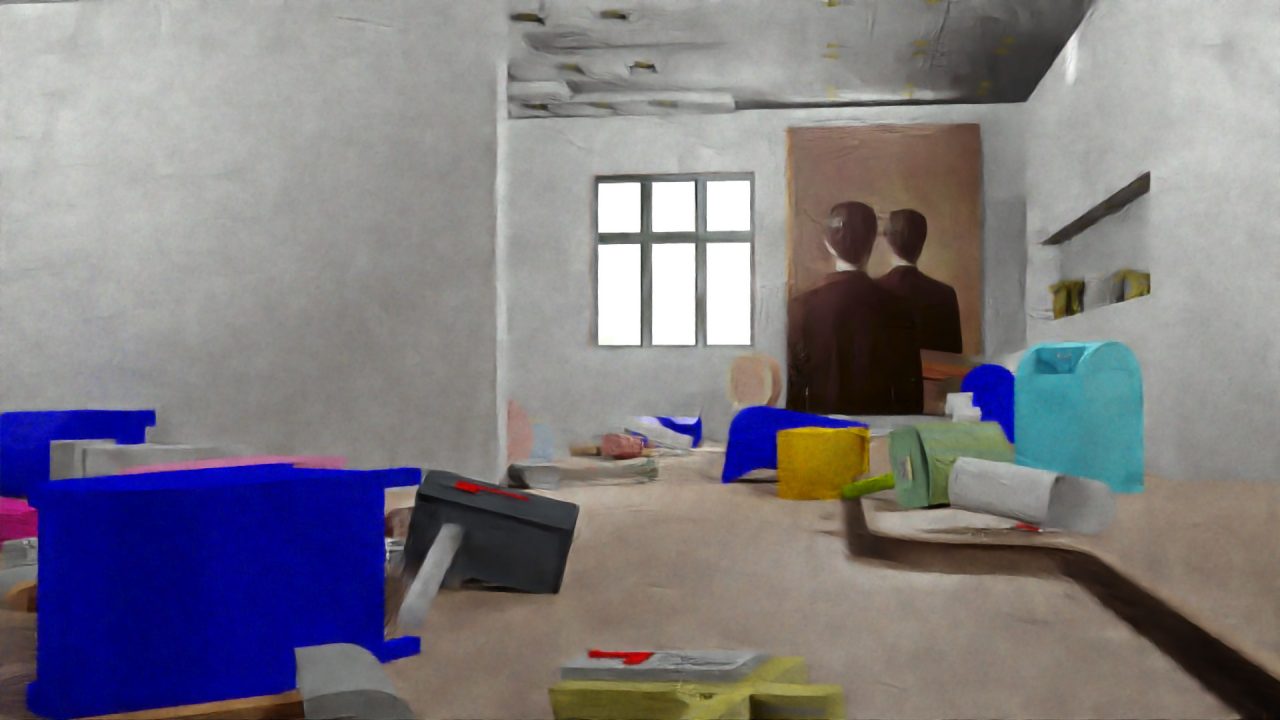
How do you know if something is a chair? What may sound like either a banal rhetorical question or a pretentious design deliberation is not irrelevant when it comes to training robots and other technologies to live with us. Simone C Niquille’s project, Regarding The Pain of SpotMini, forms the basis for a 3D animation titled HOMESCHOOL, one of the five projects that make up the Housing the Human design prototypes program. It demonstrates how the unwitting biases embedded in the forms and standards of our designed environments are revealed through technological glitches.
SpotMini is “a nimble robot that handles objects, climbs stairs, and will operate in offices, homes and outdoors,” reads the website of developer Boston Dynamics. Boston Dynamics is a commercial spin-off from research conducted by founder and CEO Marc Raibert at MIT in the 1990s into quadruped robots. Its dog-like robot Spot and its domestic pup SpotMini have over the past four years amassed millions of views on YouTube, where videos show them climbing stairs, dancing, pulling heavy objects, and walking in the woods. A video showing SpotMini opening a door while thwarted by a hockey stick-wielding engineer is what first drew Niquille’s attention.
“The videos strike a peculiar tone between slapstick comedy, meme-worthy content and engineering test footage,” Niquille writes in an essay based on her initial visual research, adding that “seeing a robot fail is amusing, yet carries an uncanny undertone.” The machine-vision algorithms of robots are trained according to datasets of what domestic and office spaces and their contents should conform to. These “shoulds” can be traced back to the standardizing models that range from formal standards such as the 15th-century origins of the mesh grid used to digitally model 3D space and 20th-century furniture sizes, to how the personal and pragmatic biases of the engineers influence their notion of the typical habits and routines of humans.
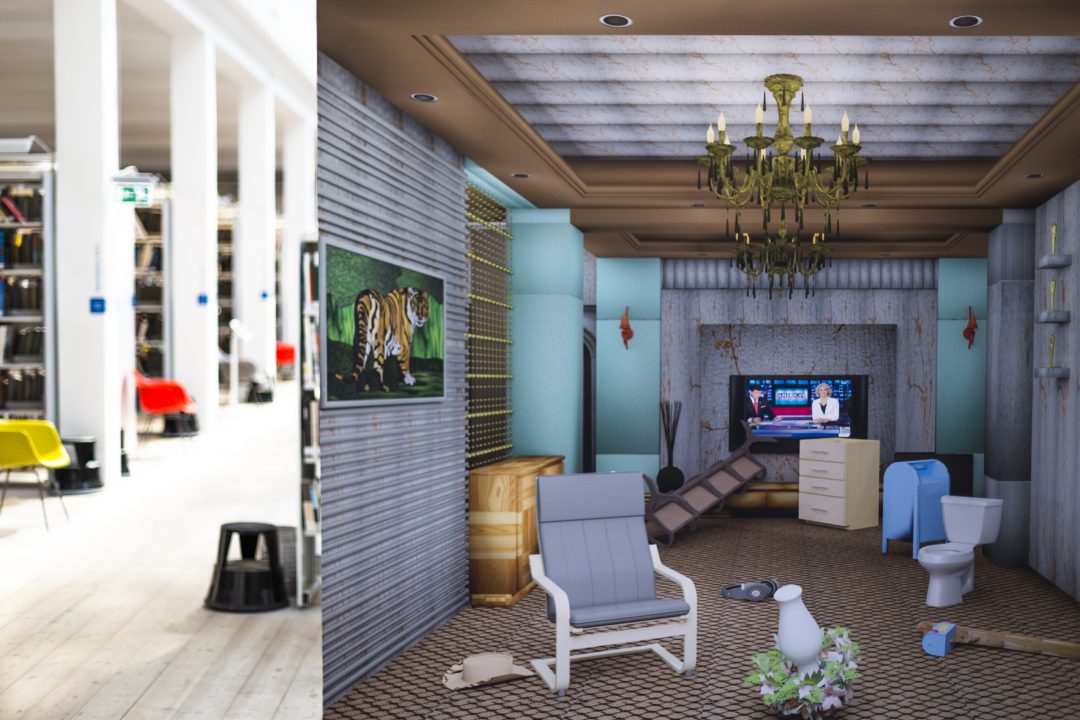
“An autonomous robot’s struggle to navigate spaces designed for humans reveals the designer’s assumptions regarding the intended user (and the robot’s failure at imitating them). Once [deemed as] functioning flawlessly, these assumptions—causing violent consequence not only for the robot but, more importantly, for the excluded user—are hermetically sealed within automation,” Niquille says, using the 2015 case of a woman attacked by a Roomba autonomous vacuum cleaner to illustrate these consequences. While the public outcry echoes the fears of the mythical robot apocalypse, what is really at stake according to Niquille, is that the woman was not behaving in the way that the Roomba had been trained to recognize: she was sleeping on the floor, not on a bed; during the day, when she should have been at work.
Never mind the question what is a chair, how does a robot know what is a human? When attempting to quantify the complexity of human behavior in sets of 0s and 1s, glitches are inevitable. The algorithm-training decisions, informed by inevitably incomplete models of human behavior, could come to imprison our behavior in conforming to models or suffer the (violent) consequences. “It’s never going to be a model of reality, but a model of someone’s reality, be it that of the engineer who puts together the training database or a model of what SketchUp or other databases of domestic environments contain,” Niquille explains.
Such conformism also advances particular commercial interests. For instance, through her design research into what the training database of SpotMini might be, Niquille identified that the majority of furniture was from IKEA. Training machines to navigate domestic spaces, which have a far higher degree of variability in furniture form and spatial layout than offices, is a lot trickier. Thus the relevance of what a chair is: “Are chairs furniture with four legs and back rests, or any object for sitting?” The answer can not only determine the degree of safety with which autonomous robots navigate spaces originally designed for humans, but predict the form that future spaces, designed around human and robot cohabitation, might take, and which commercial partners get to decide.
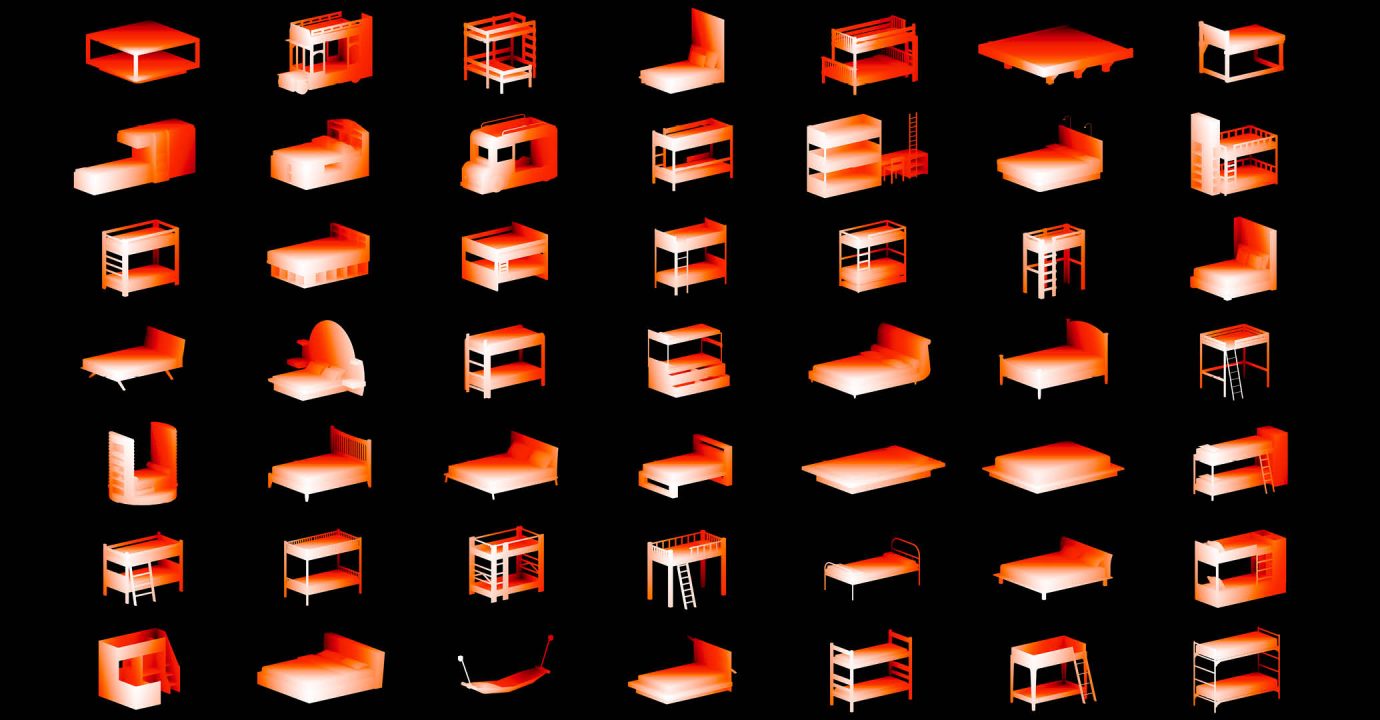
It is precisely these implications that motivate Niquille’s design research practice, which seeks to “make visible the thing that is always invisible once you’ve created a computer vision algorithm—the training data.” First studying graphic design at Rhode Island School of Design before completing a Masters in Visual Strategies at Sandberg Instituut, Niquille’s methodology uses available online data to reconstruct and thereby reverse-engineer the original dataset. “These constructed images embedded in training databases become ‘objective reality’ and the model for domesticity, even though they can’t be because we can never amass enough data to encompass the complexity of real life.”
After reverse-engineering the Boston Dynamics test house using SpotMini videos and interviews with the developers, Niquille’s research expanded to include similar databases that model human spaces such as the CNET database, which is “comprised of around 100,000 3D objects and a bunch of different room layouts, organized in categories such as living room, bedroom, office and kitchen.” Using these layouts and objects as assets, Niquille is designing a film character that sparks reflection and discussion about whether we humans design our technology or our technology designs us.